Collaboration with impact
Advance an exploratory research project that you care about, deepen your company’s future hiring pipeline, or simply feel good about contributing to the education of the next generation––all with an investment of 30 minutes/week.
Over the past eight years, participation in the Center for Data Science and Artificial Intelligence’s Industry Mentorship Program has yielded numerous interesting projects, many publications, and a few awards. Previous industry participants include Google, Amazon, Microsoft, Meta, DeepMind, IBM, Adobe, Oracle, Bloomberg, CZI, ISI, Voya, GE Health, as well as startups. Consider participating this coming spring.
Program
The Industry Mentorship Program matches small teams of Master’s students trained in Data Science and AI with an industry-proposed project.
Our program provides industry partners with cutting edge AI and data science solutions while allowing Master’s students to hone their skills by working on real-world projects.
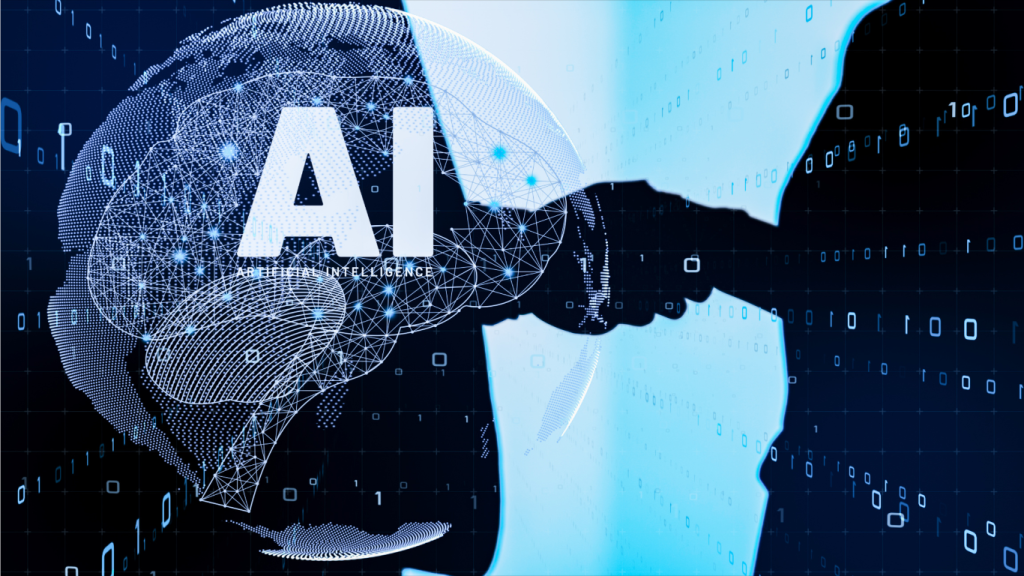
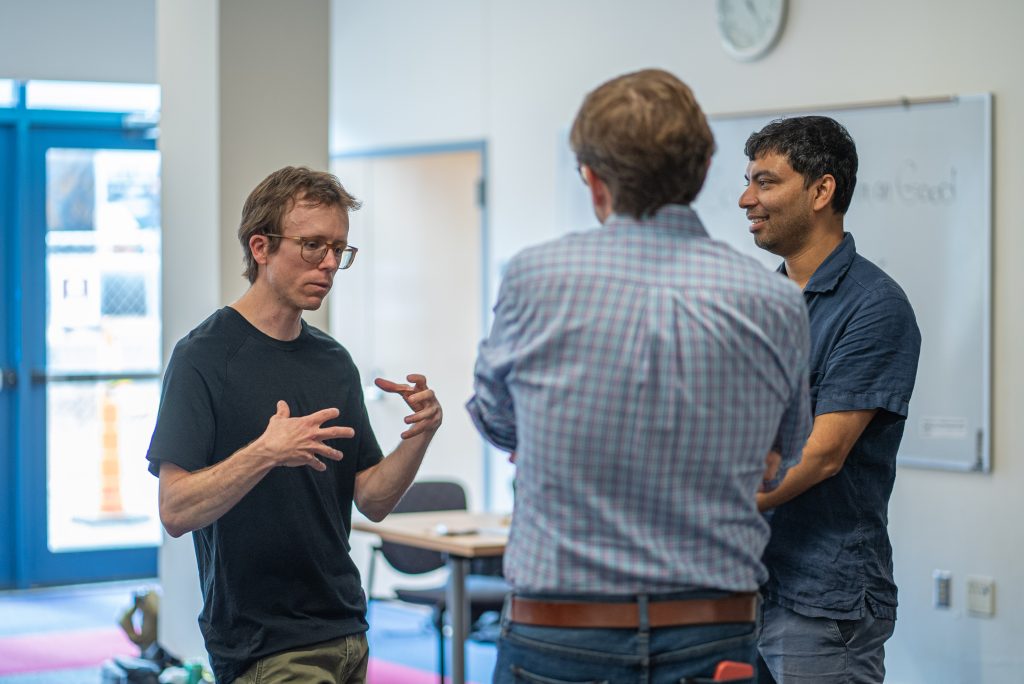
Our Partners
By sponsoring a project, our industry partners gain access to extensive University resources and expertise from our talented faculty, PhD, and Master’s students.
Projects have led to publications in prestigious journals, production prototypes, and production quality code, while also providing ample brand development and recruitment opportunities.
Interested potential partners are strongly encouraged to reach out to learn more at any stage of project development or readiness to engage with our program.
Students
Through close collaboration with industry partners, PhD student advisors, and faculty experts, students develop industry-relevant skills, gain networking opportunities, and expand their data science expertise while working on highly relevant problems. The mentorship program has led to a number of publications in top tier journals in addition to job and networking opportunities with industry leaders.
Master’s students in the data science concentration who have completed at least two core courses are invited to apply for admission to the course (COMPSCI 696DS). Look out for an email in mid-October with instructions on how to apply for the course, which is offered every Spring.
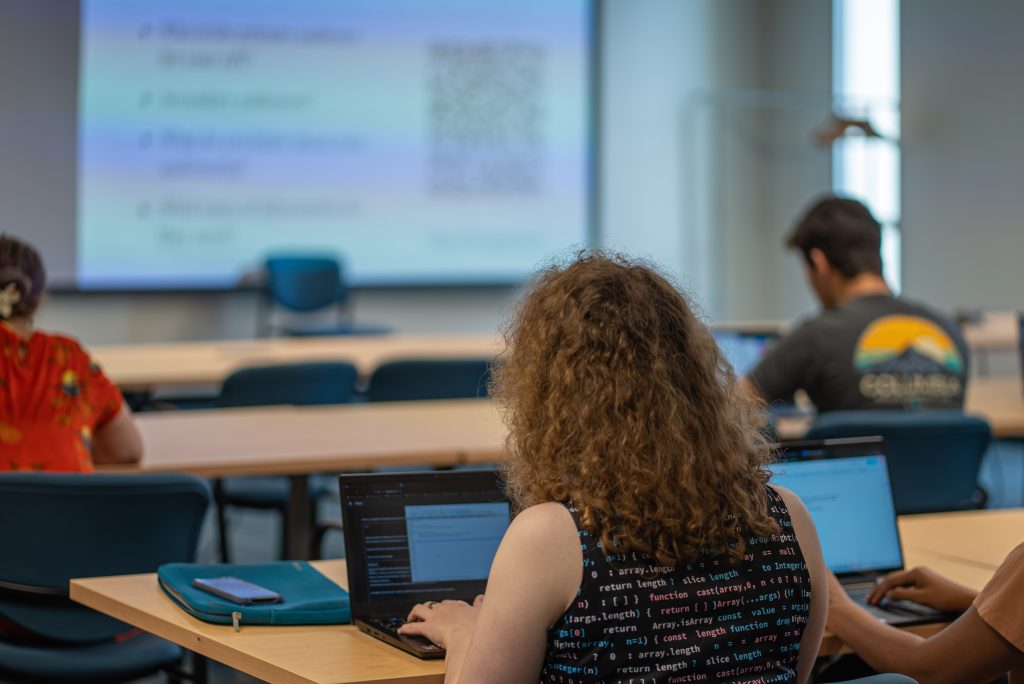