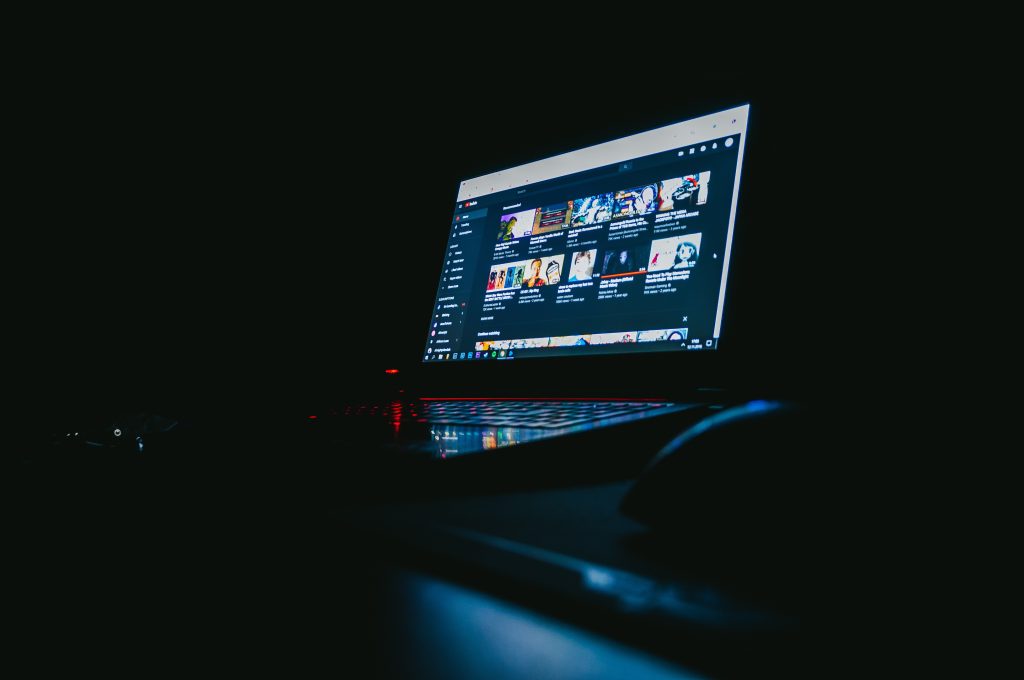
Partner: Media Cloud
DS4CG 2023. With the current surge in multimodal media shared online, particularly from platforms like YouTube and Instagram, the need for multimodal hate speech detection systems has grown. We created an evaluation dataset of YouTube videos from specific types of content creators and compared how features from the audio and transcribed text in a video can be used to flag extreme speech using machine learning.